How are LLMs like ChatGPT going to revolutionize real estate?
Here is how AIs like ChatGPT are going to revolutionise real estate: invisibly, by increasing efficiency in the market, and visibly, by making customer services a bit cheaper and higher quality.
AI is already in real estate, and has been for a while, but it’s not AI like ChatGPT. The AI in real estate is a collection of technology and techniques known generically as Machine Learning (ML) and it uses the same basic building blocks, called neural networks, that ChatGPT uses, but with different structures and different arrangements of the building blocks because they have a different purpose to ChatGPT.
Trulia is an example of a proptech company leveraging the power of ML. They’ve used it to build their property recommendation engine, for predicting user engagement, and for identifying property features from photographs.
These are things ChatGPT can’t do. ChatGPT is a Large Language Model (LLM). It’s a multi-layer neural network that has been fed a tremendous amount of text and it is very good at stringing words together into sentences in response to some input text.
It has been fed so much text that it is very good at masquerading as an under-trained lawyer, a sub-par business analyst, a mediocre marketer, a hack journalist, etc, simply by stringing together pieces of the text it has been trained on.
While being very good at generating text, LLMs like ChatGPT aren’t very good at math. You can’t use them to do things like predict user engagement or identify property features from photographs.
So what are LLMs like ChatGPT good for?
What LLMs are good at is bridging the vast gulf between human written text and the kind of numerical inputs ML systems can deal with.
This can be as simple as basic sentiment analysis, turning a review like “This is so not the best hamburger joint in town” into a -1, and a review like “I hate how good their fries are” into a +1 – simple numbers that can be used in a prediction model.
Stepping beyond this, LLMs can be used to turn text into ranks. An LLM can consistently turn, say, countertop descriptions like “mustard yellow formica”, “granite”, “hand-poured, hand-polished concrete” into a range from 1 to 10. The real value in this is that when it sees a new description, like “clear resin featuring embedded seashells”, it can fit it into the same range.
Moving beyond simple ranking, we can combine it with data extraction. Here is an example where an LLM pulls specific data from a police report. It could just as easily be a property listing:
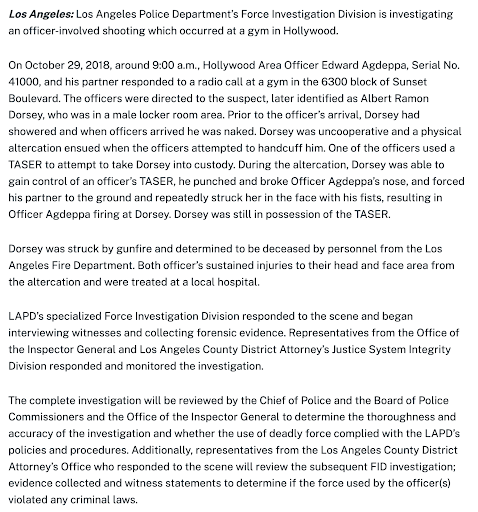
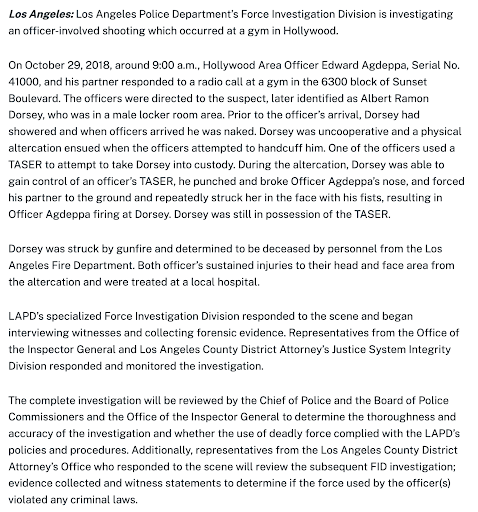
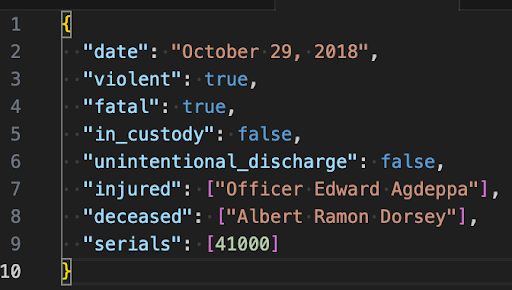
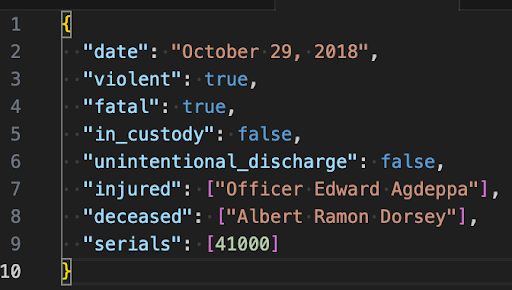
This kind of “encoding” has normally been a job for humans. This drove up the price for the production and updating of useful databases, making them expensive, rare and proprietary. Spending the money and the time to do the encoding and compile a database was a nice way to build a moat.
But now it’s not a moat because anyone who can connect a web scraper (or web scraping service) to an API call to an LLM can fill a database with any information they want in days for next to nothing.
So getting data isn’t going to be much of a problem any more. That’s going to bring efficiency increases. Maybe some parts of the market will eke out a few more percentage points in returns, but only if they can use the data.
Trulia, for example, isn’t running their services on LLMs. LLMs will only be part of the pipeline. The real work and real value will still be done with classic ML models fed with higher quality data encoded by LLMs.
So, that’s the invisible part of AIs impact on real estate. Let’s now look at the likely visible impact.
AI, LLMs and email and chat
This visible impact is going to be based on the highly visible features of LLMs – their ability to generate text and respond in a conversational manner.
We’ve already seen too many real estate listing generators that use LLMs. That last link, agently, provides LLM generated property descriptions as one of their less important features.
This is one of the recurring gotchas of building products with LLMs. It is so easy to get text out of an LLM that whatever product idea you have, if it is just producing text then your business model is in danger of becoming another company’s bullet point.
Is AI Chat going to take over customer comms?
Chat is the big, consumer-facing application of LLMs like ChatGPT, Bing and Bard. With the ability to train LLMs on your business’s documentation via fine-tuning (OpenAI’s page on it) or using embeddings to constrain the information an LLM can work from, it seems we can all turn over our customer enquiries to software, saving us huge sums of money.
If only it were that simple.
The problem with using LLMs to “chat” to your customers is that you need to rely on raw text from the LLM. LLMs don’t have any judgement. They just generate text. The result is that they “hallucinate” – produce wrong or even wildly wrong responses – and there is no way to guard against this except having a human in the loop.
If you are building your customer service chatbot on top of an LLM like GPT-4, even with fine-tuning, the data you supply (chat logs, FAQs, blog articles) will be such a tiny percentage of the LLMs “knowledge” that it will inevitably “hallucinate” when answering questions. It might even insert information from a competitor’s products (that were documented on the internet prior to September 2021).
The other problem is that prompt jailbreaks – strategically written text that tricks the LLM into leaking information or hijacks it for the user’s own purposes – are not a solved problem.
The end result is that LLMs are not predictable enough to be trusted and so are not going to replace staff in customer service roles. Instead, they are going to act as an assistant to them. They’re going to help customer service staff find answers faster, they’re going to help customer service staff provide better answers, and they’re going to help customer service staff manage complex and ongoing interactions.
Crisp wrote up a fantastic deep dive into their efforts to add AI to their customer service. It’s not easy. It’s not cheap. It will get cheaper, but when you look at the steps they had to go through it is hard to imagine it getting easier.
Zendesk, the customer service SaaS, have also found that AI and LLMs are going to augment staff, helping to improve customer experience, instead of replacing them.
So AI chat as it is popularly imagined – replacing customer service staff with a bit of code – is not going to happen any time soon. For anything other than the most basic and pro forma customer enquiries, where an LLM chatbot might be limited to being a high-powered FAQ regurgitation engine, humans will still be in the loop and on the payroll.
What’s Proptech going to do with AI?
That’s the big question. The AI services landscape is already fracturing into service providers who will run LLMs for you, fine-tune them for you, host your data for you, etc. AI tools are generic tools. At the coal face they work just as well for any industry, be it real estate or livestock management. So the shovel sellers are already busy selling shovels.
Proptech startups need to be looking at what untapped data is out there, preferably in text format if you want to jump on the LLM bandwagon. That text could be in paper format. Have you noticed how good OCR has gotten in the last year or two?
The data might already be sitting on your server. A database of transactions is a prediction model waiting to be trained. Prediction gives you the opportunity to optimise and de-risk, two things every industry wants to pay for.
Whether you want to reduce the cost of comms with tenants or streamline nine figure real estate transactions or improve compliance in building maintenance schedules, you need to be asking yourself where you can scrape, collect, licence or buy the data you need.
That, we expect, will be easier to do than hire the data scientists you’re going to need to make it work. Maybe the shovel sellers will fix that for us.